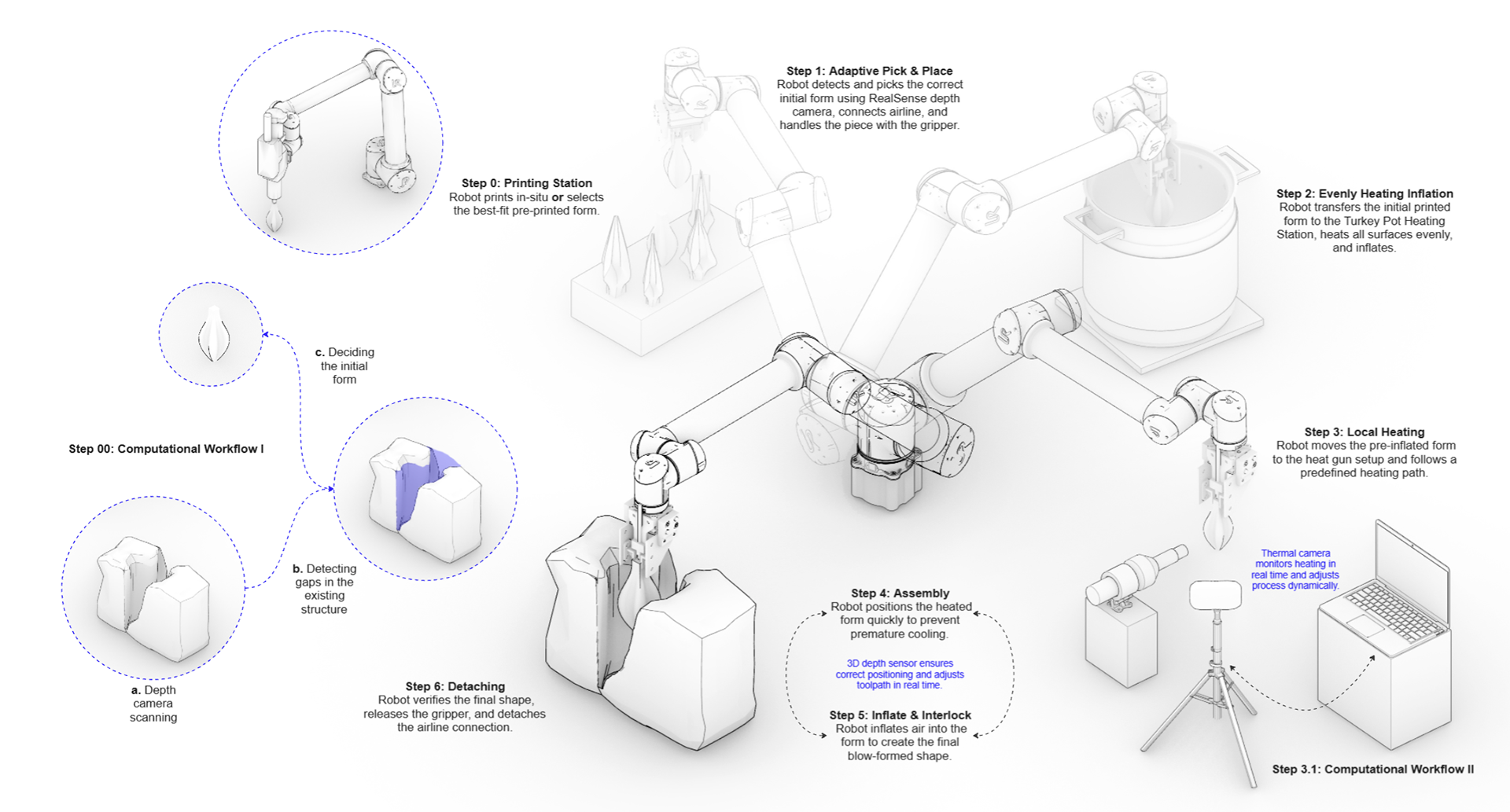
Hybrid Methods for Programmable Thermoplastic Inflation: Pre-form Encoding for In-Situ Customized Blow-Forming in Automated Surface Repair and Temporary Spatial Interventions
This research explores a blow-forming process that leverages sensor-driven feedback and robotic-assisted fabrication to dynamically shape PETG, eliminating the need for predefined molds. By integrating real-time thermal imaging and adaptive material control, the project aims to develop a precise yet flexible approach to in-situ plastic assembly, enabling scalable, efficient, and responsive fabrication for surface repair, void filling, and temporary spatial interventions.
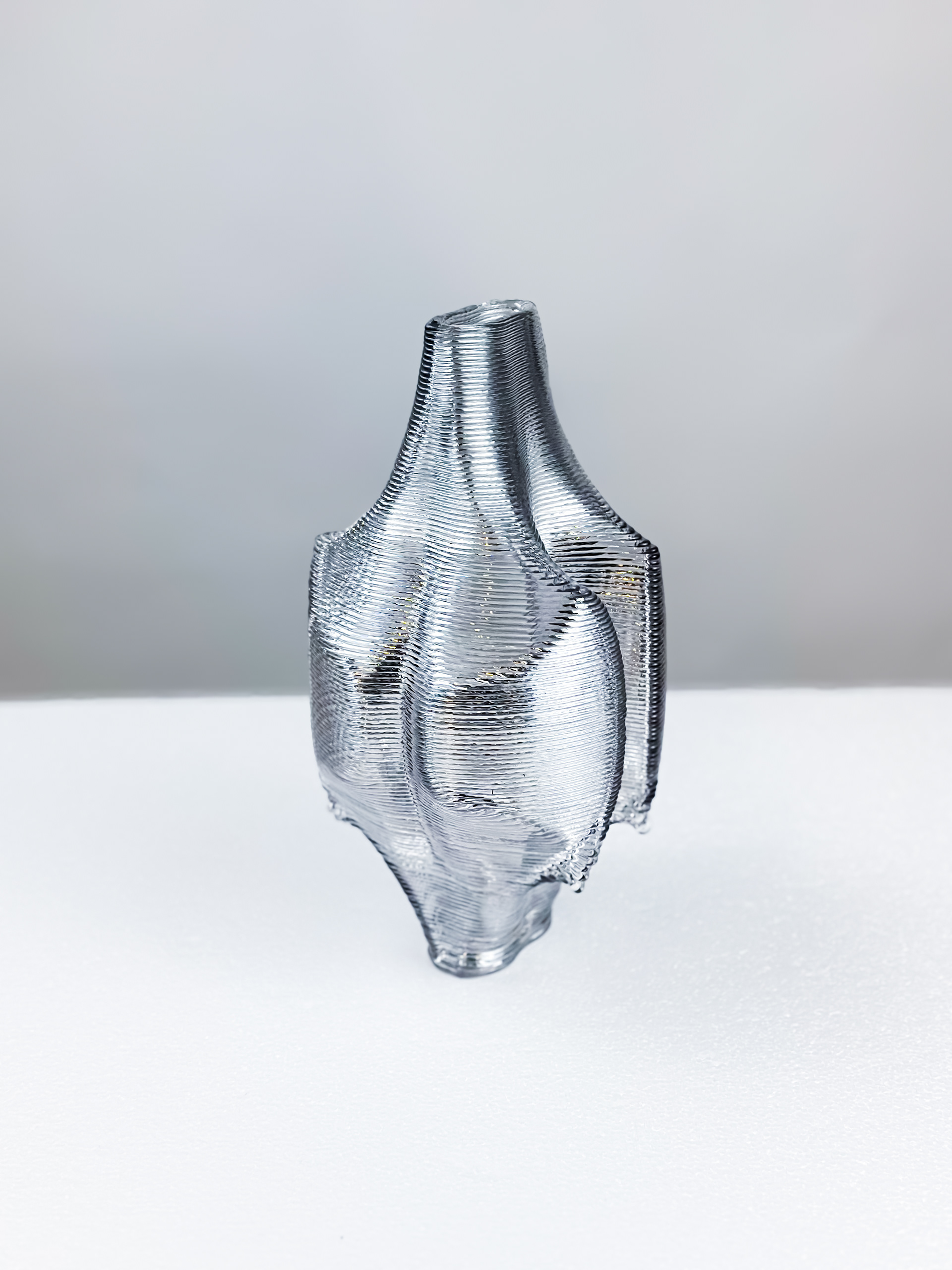
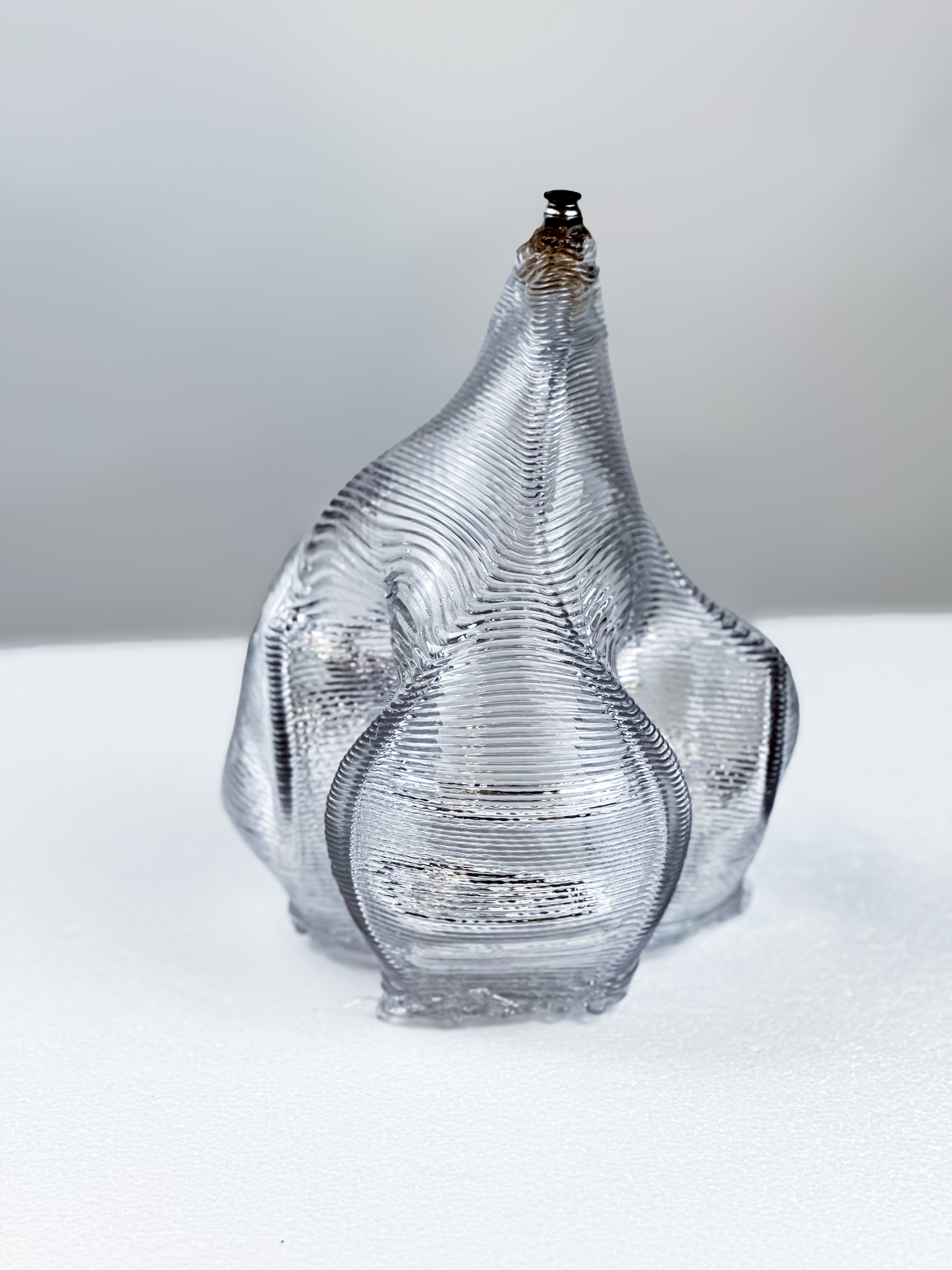
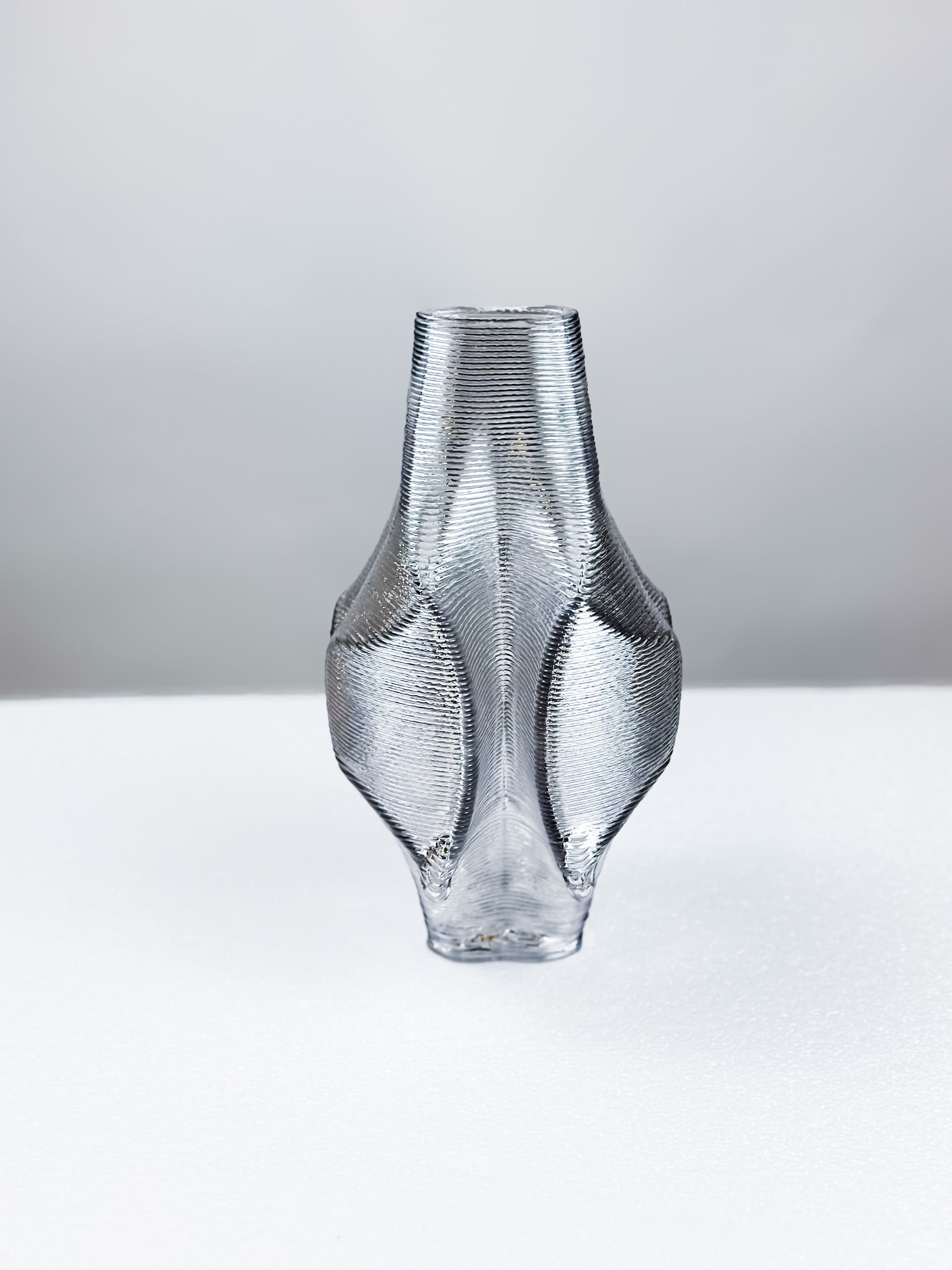
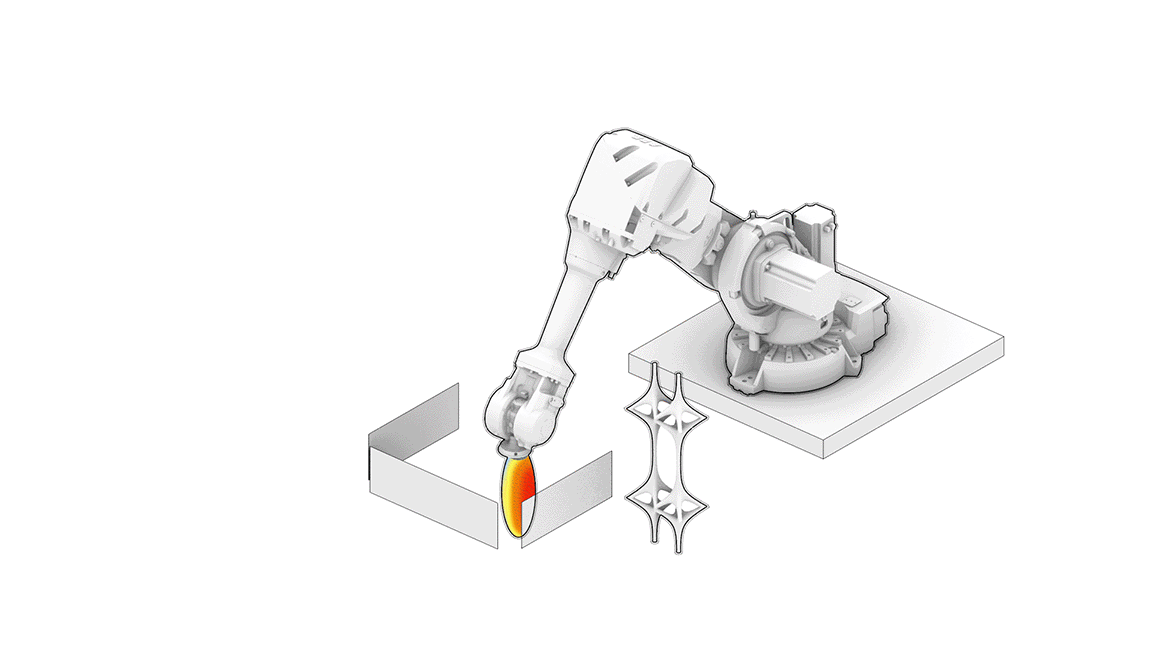
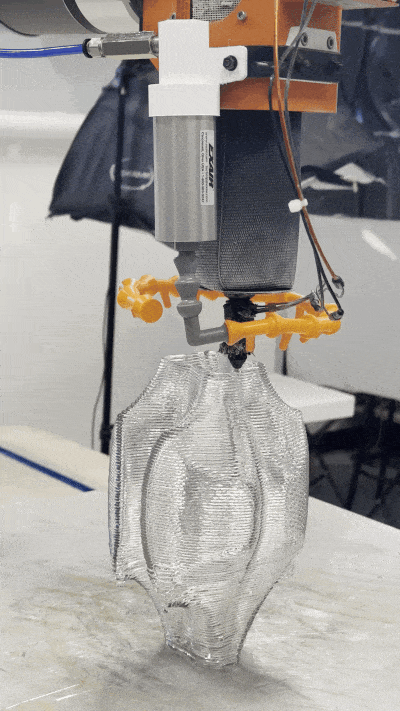
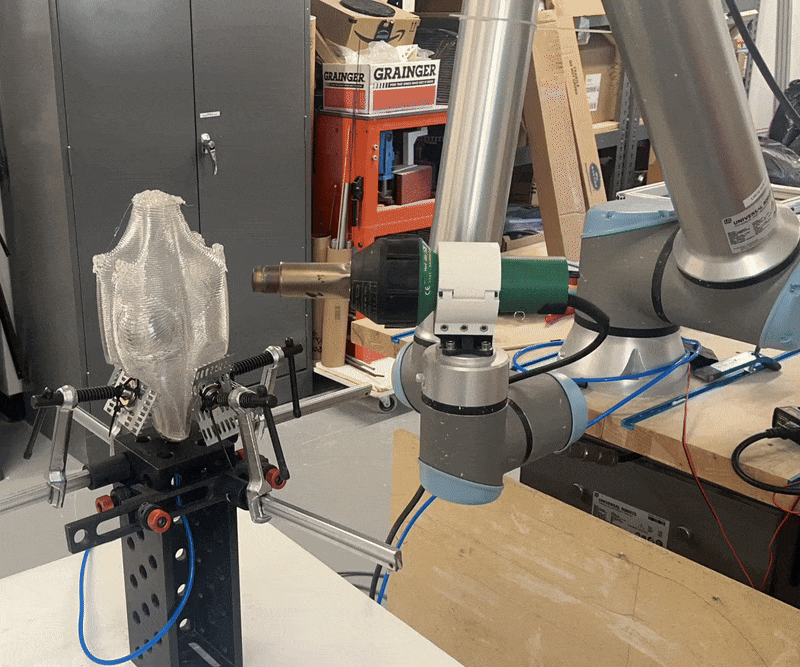
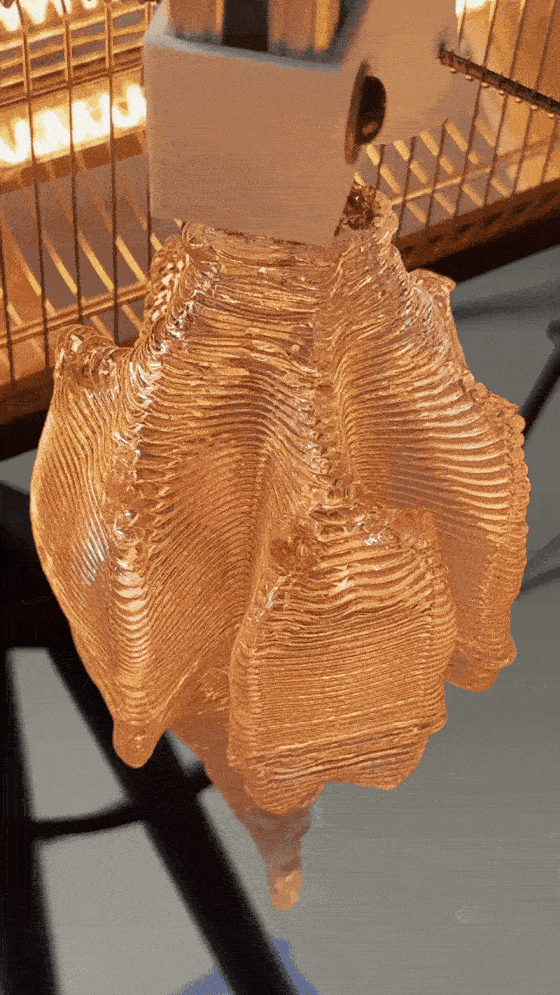
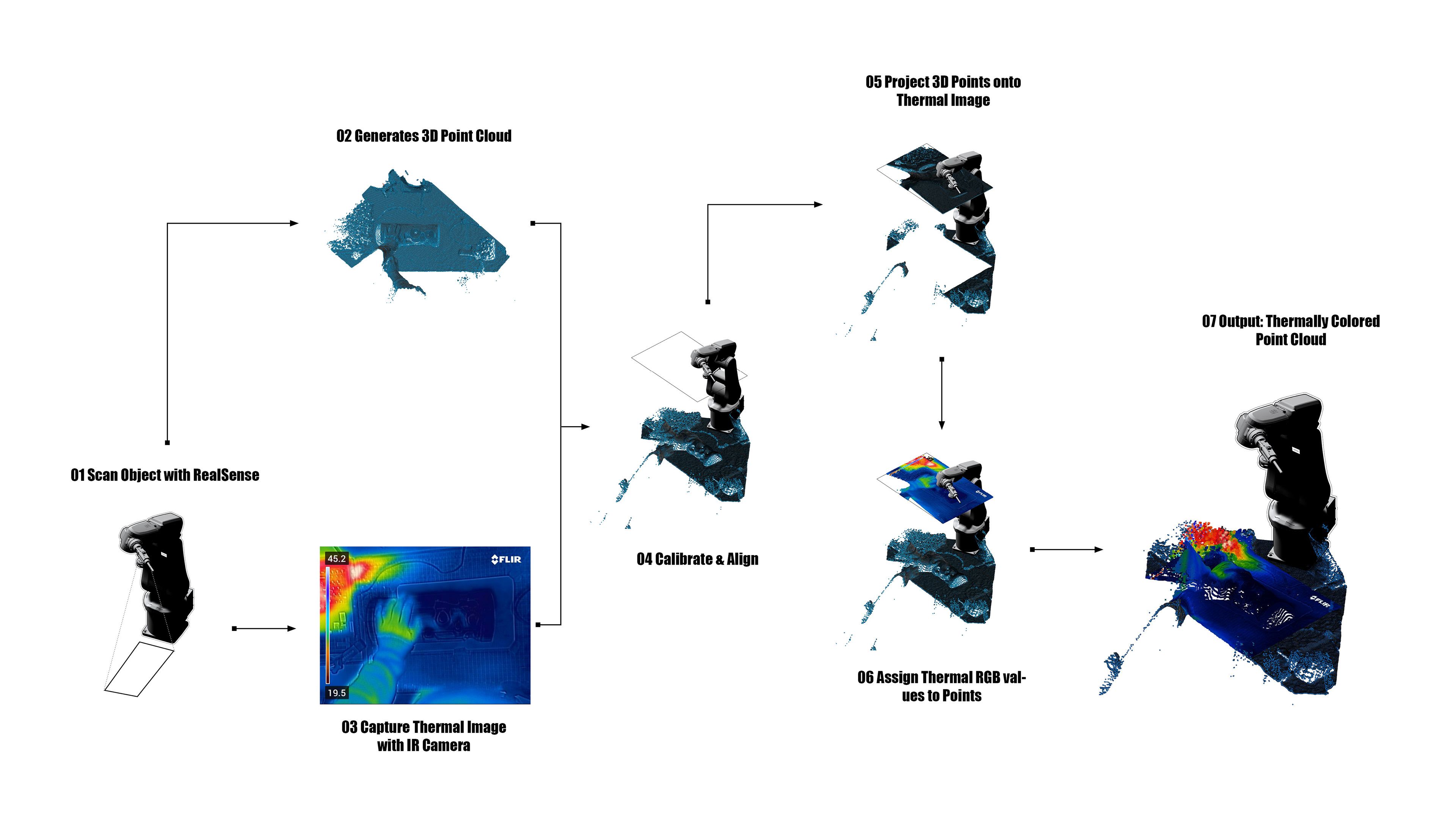
Adaptive construction for architectural and industrial applications requires real-time adjustments that traditional fabrication struggles to balance in both prescribed mold-based methods, and inaccurate freeform shaping. Existing methods for gap-filling, surface repair, and temporary enclosures rely on predefined molds, making them impractical for dynamic environments.
This research introduces a robotics-assisted blow-forming process that enables dynamic material placement for automated surface repair, void filling, and temporary spatial enclosures. By integrating real-time depth sensing, volumetric prediction, and sensor-driven thermal control, the system detects and fills gaps, cracks, and voids in real-time, eliminating the need for external molds and minimizing scanning requirements. The process uses evolutionary algorithms embedded with fabrication metadata to generate and optimize the initial 3D-printed pre-forms, ensuring programmed transformation to the final inflated forms.
A custom robotic tool equipped with an airline connection and gripper handles the pre-programmed printed PETG pre-forms, moving them between dedicated heating stations for controlled global and local heating before final placement and assembly. This sensor-driven workflow reduces the labor-intensive process of precise 3D modeling, offering a scalable and dynamic, automated alternative to conventional prefabrication methods.
This study demonstrates how evolutionary algorithms can encode adaptive fabrication methods to bridge the gap between digital simulation and non-prescribed construction methods. The findings contribute to advancements in robotic-assisted construction, proposing a new class of deployable structures based on thermoplastic inflation, adaptive manufacturing, and sustainable material applications that minimize waste through targeted heating and inflation, enabling recyclability of PETG. It offers efficient fabrication workflows that reduce modeling time, support on-site responsiveness, and optimize material use, resulting in customizable and scalable solutions for dynamic, real-world environments.
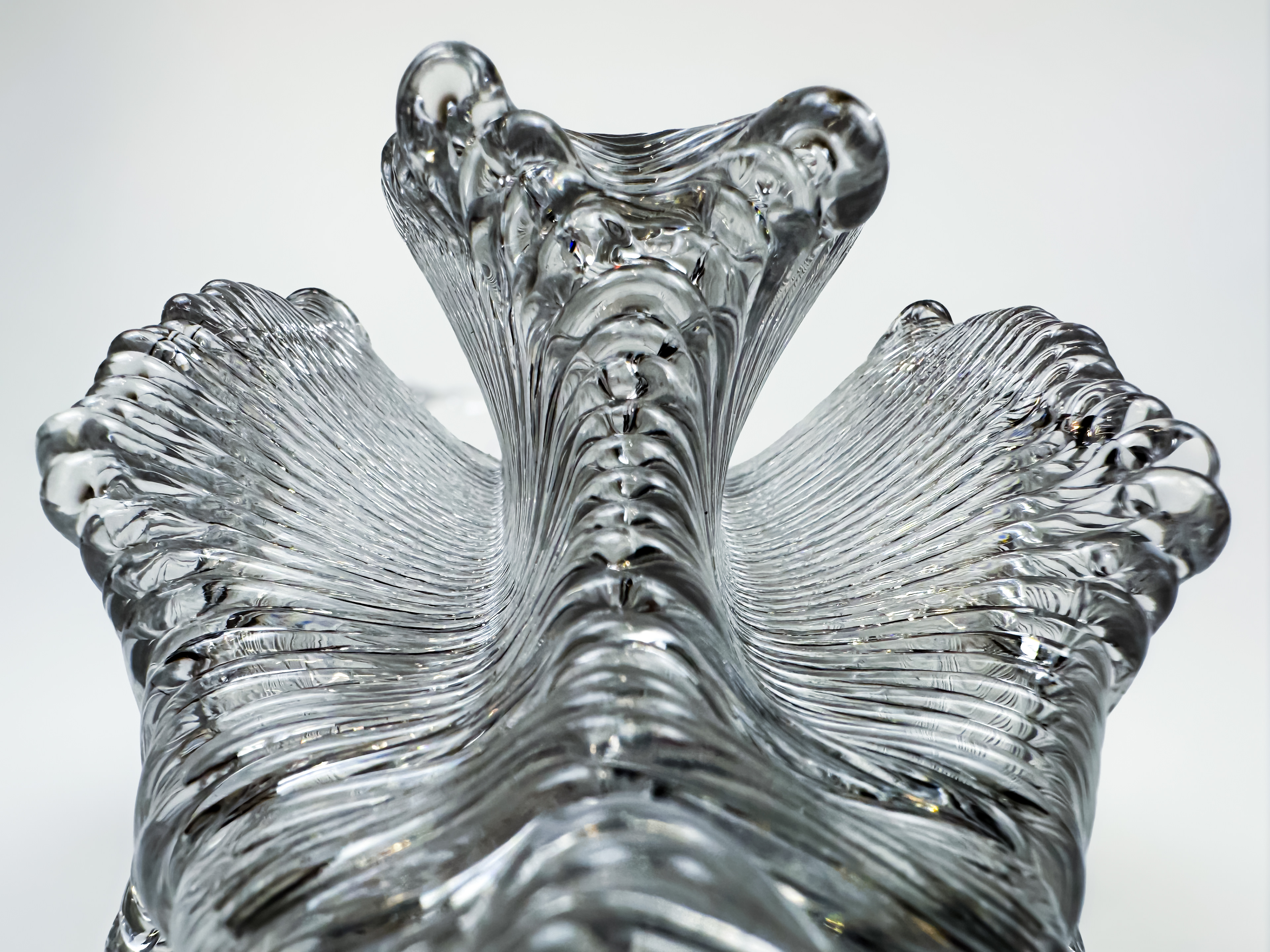
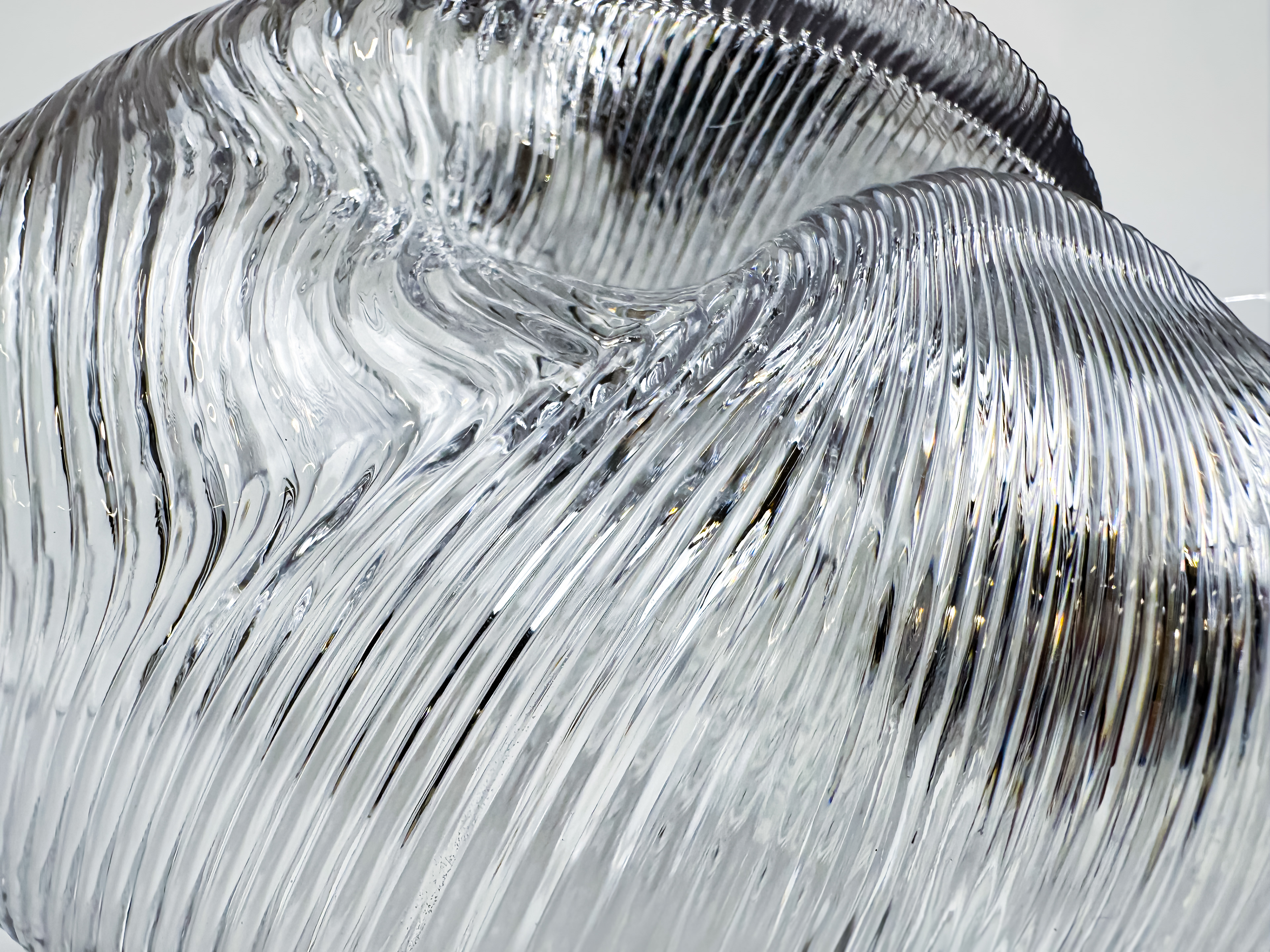
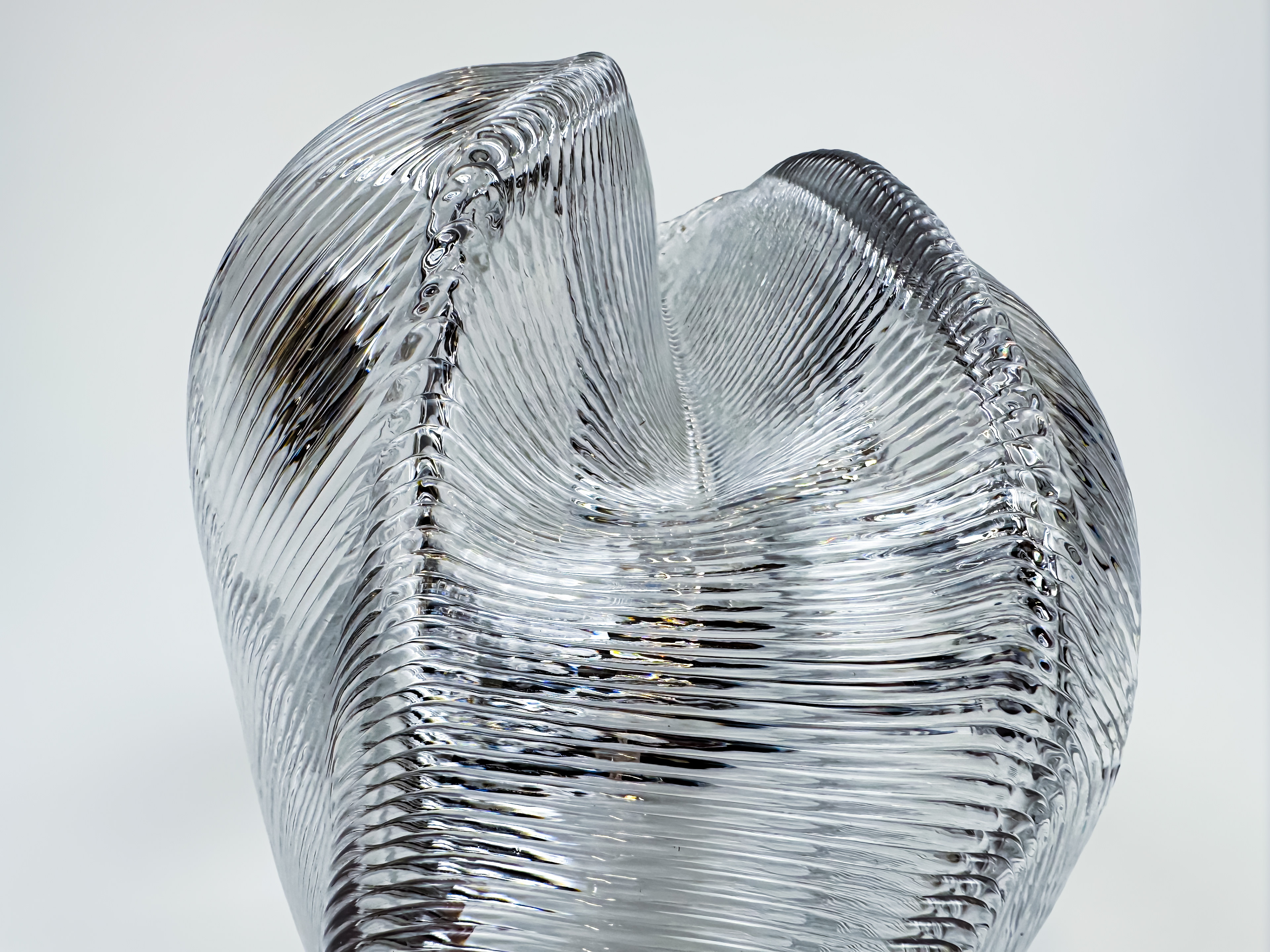
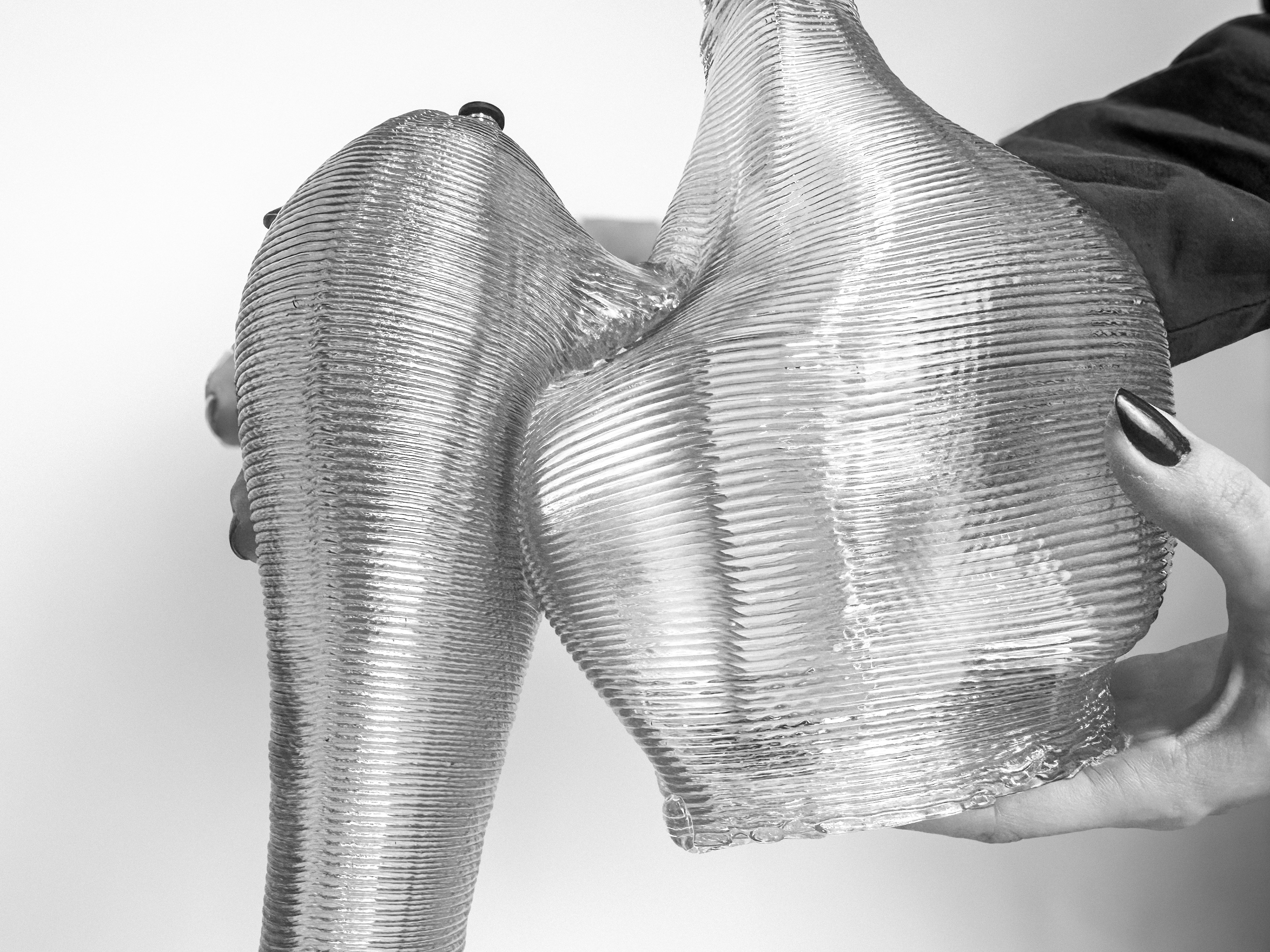
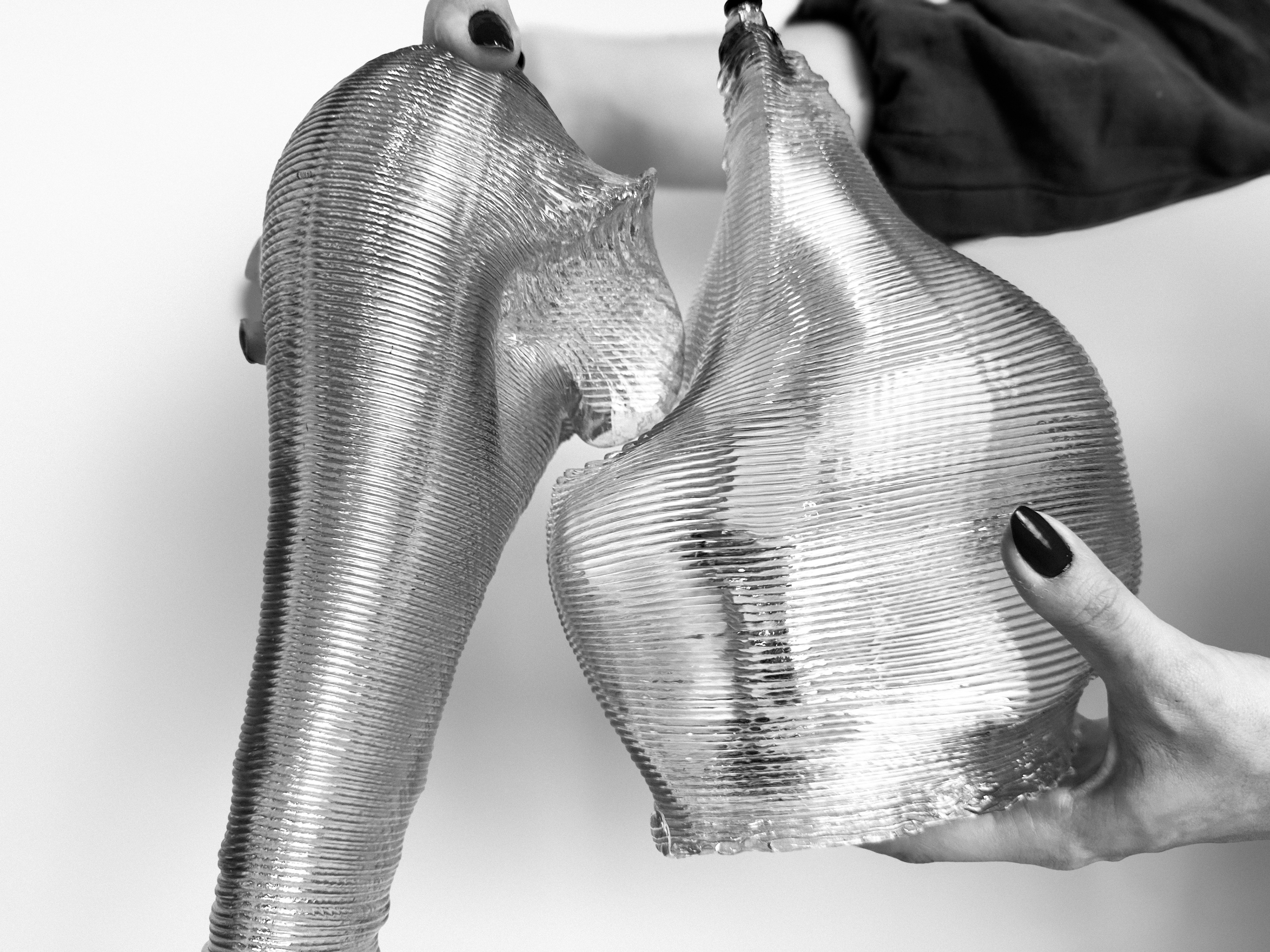
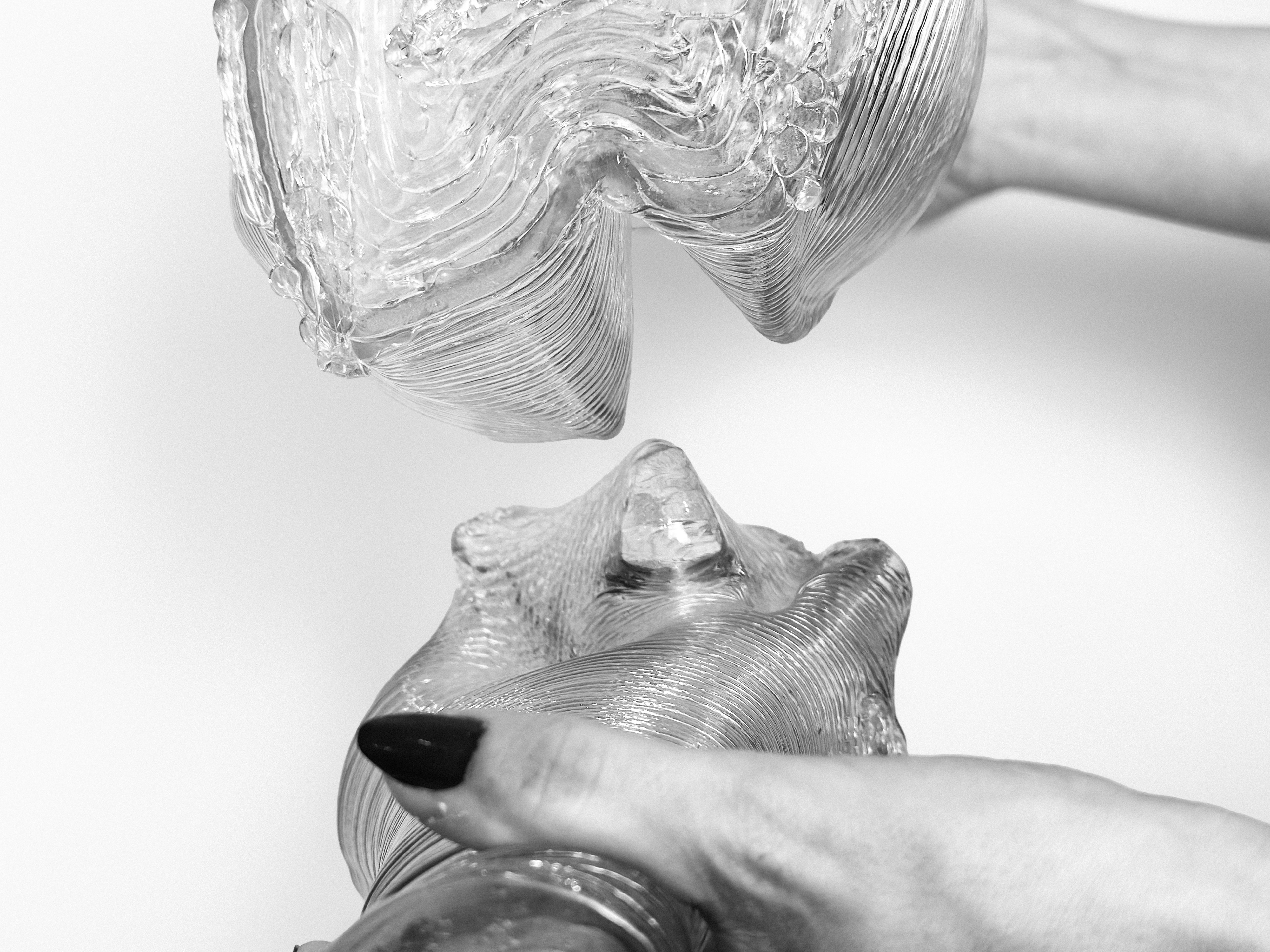
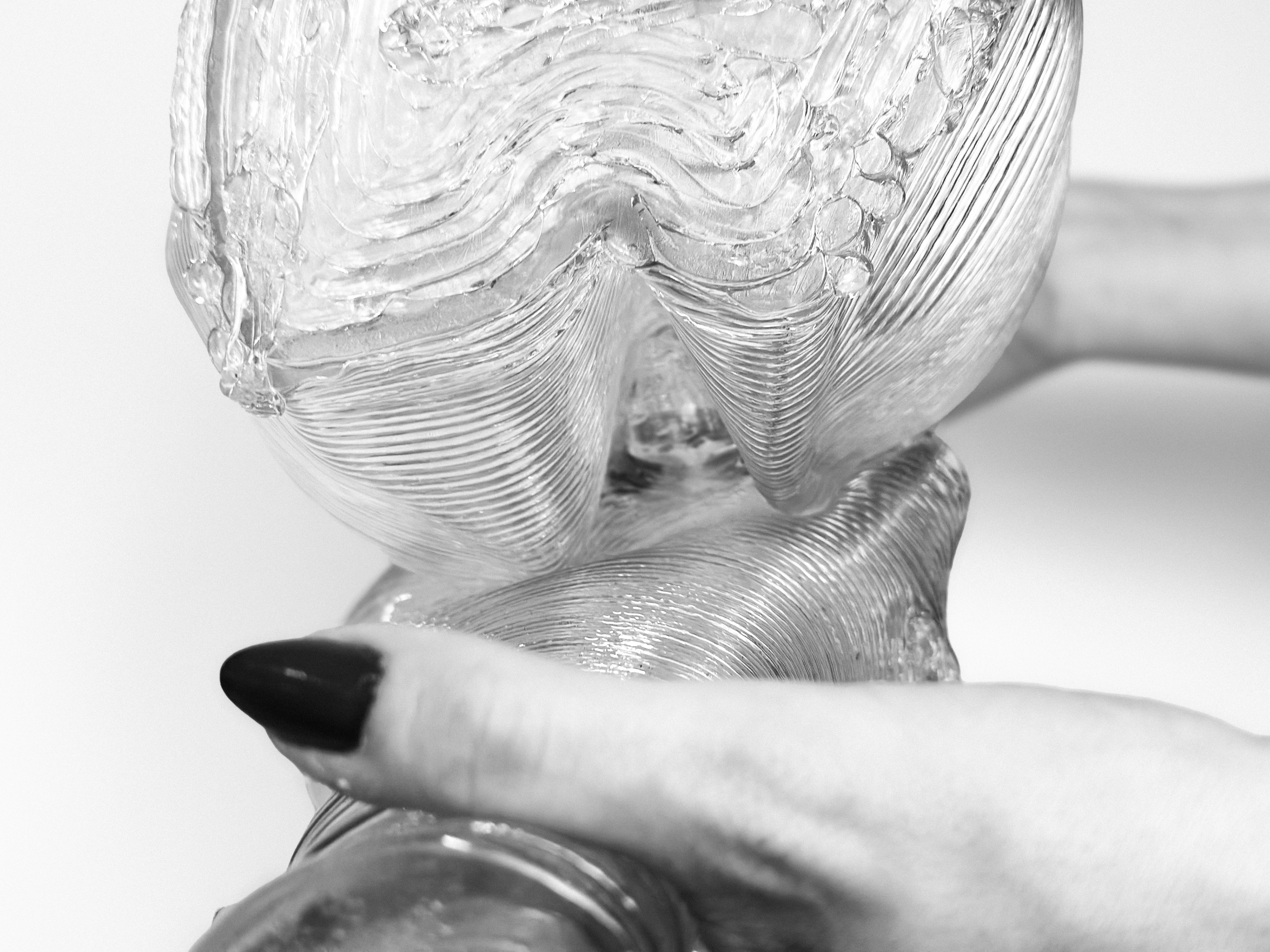
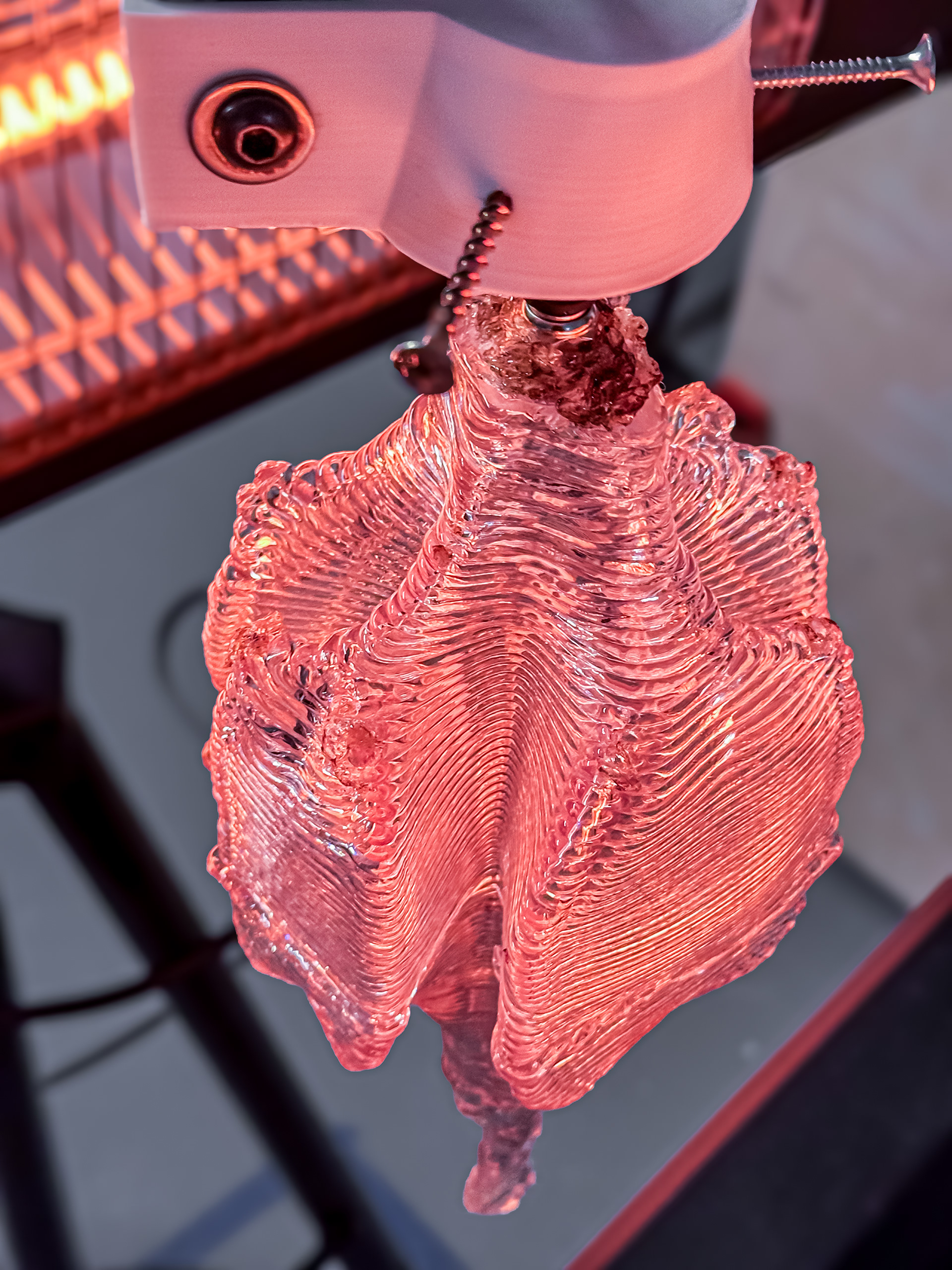
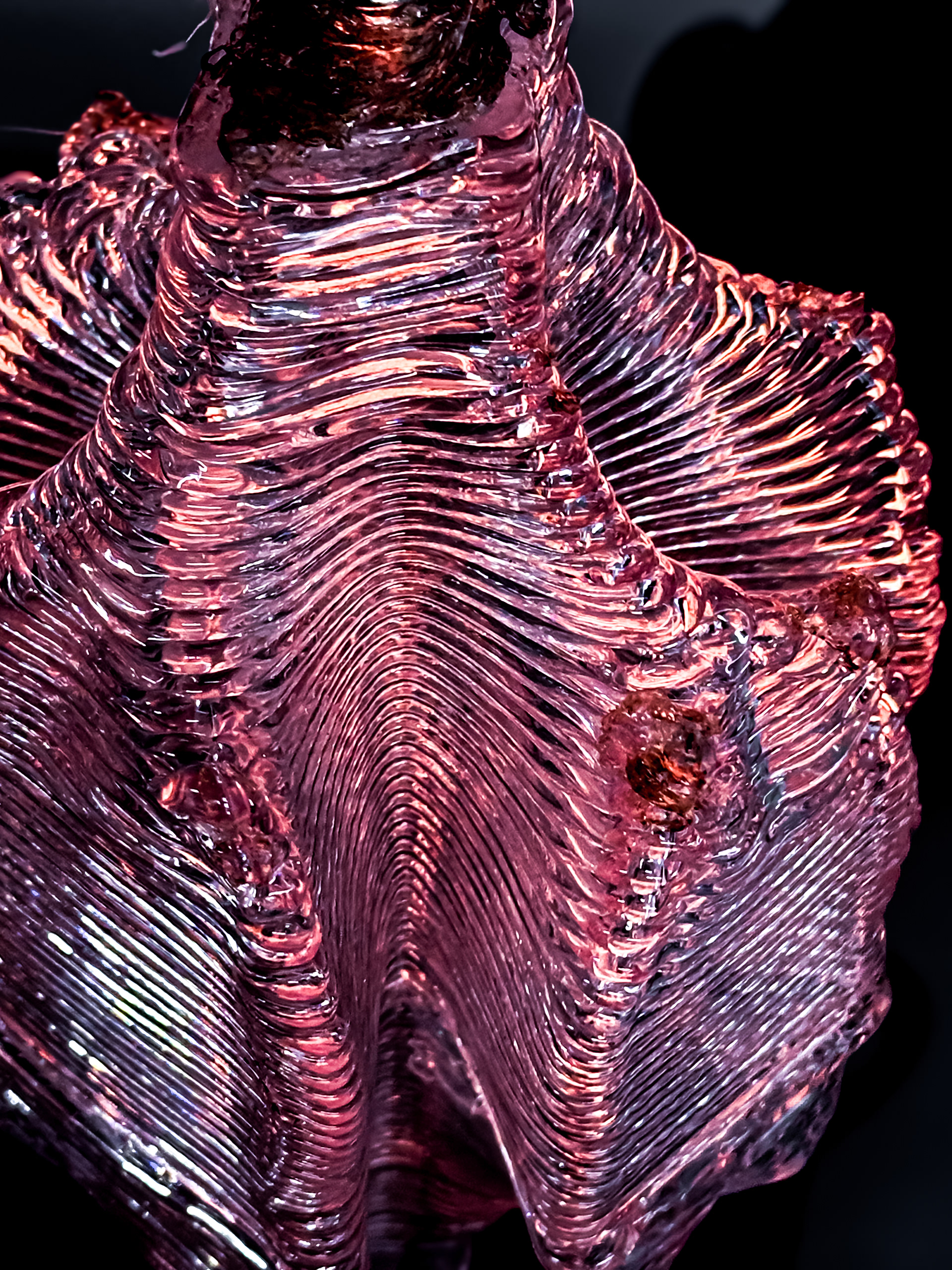
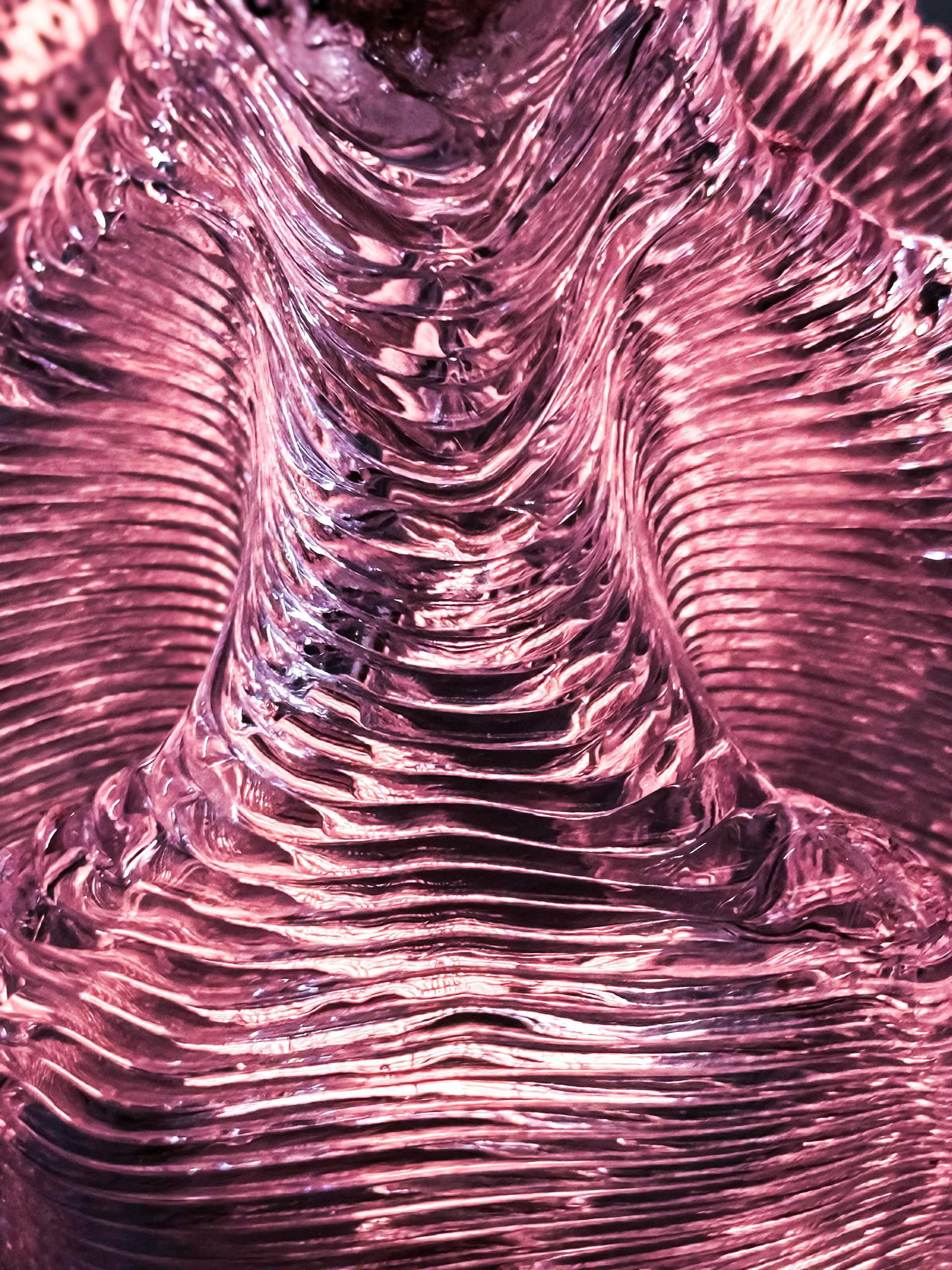
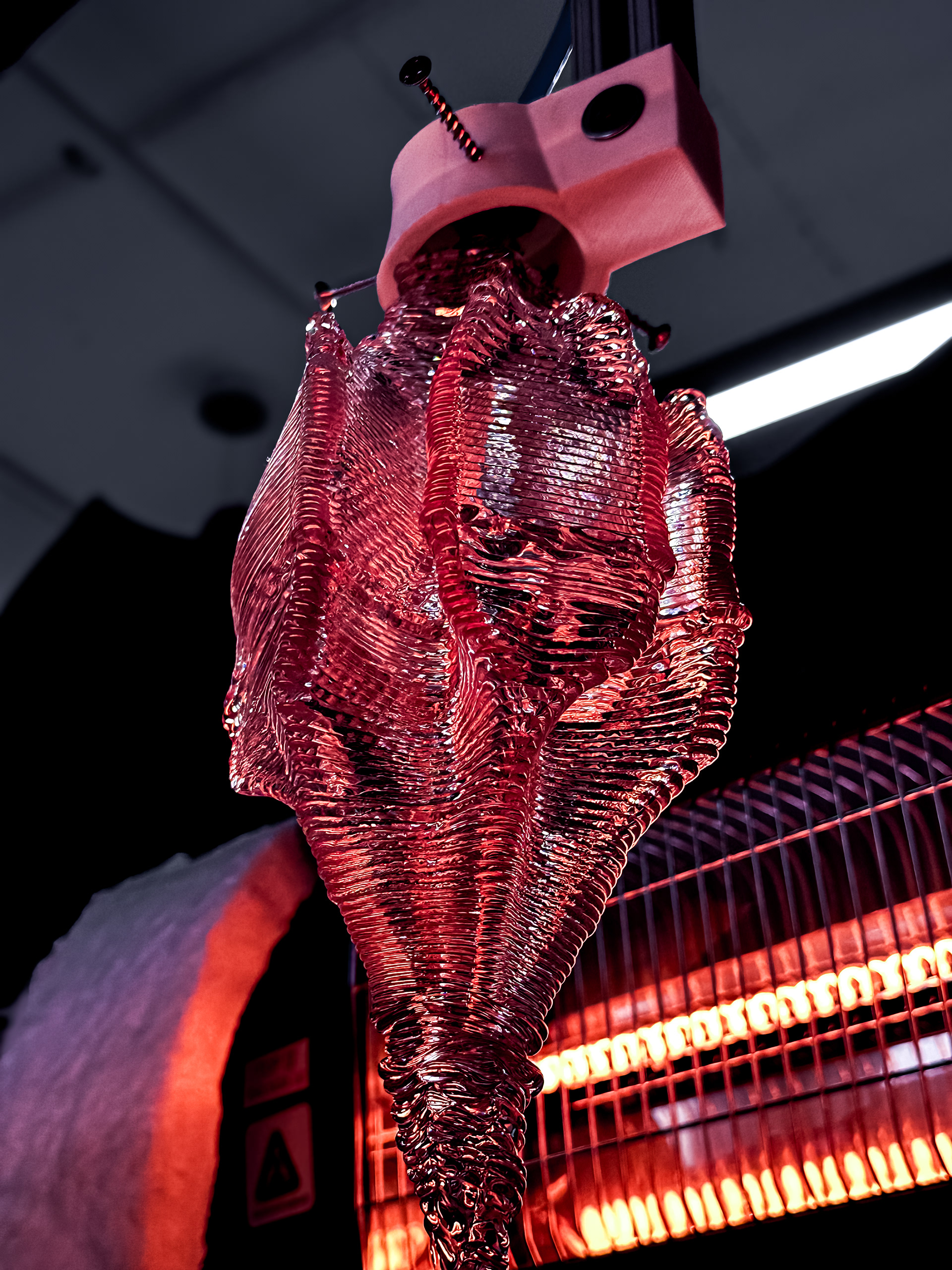
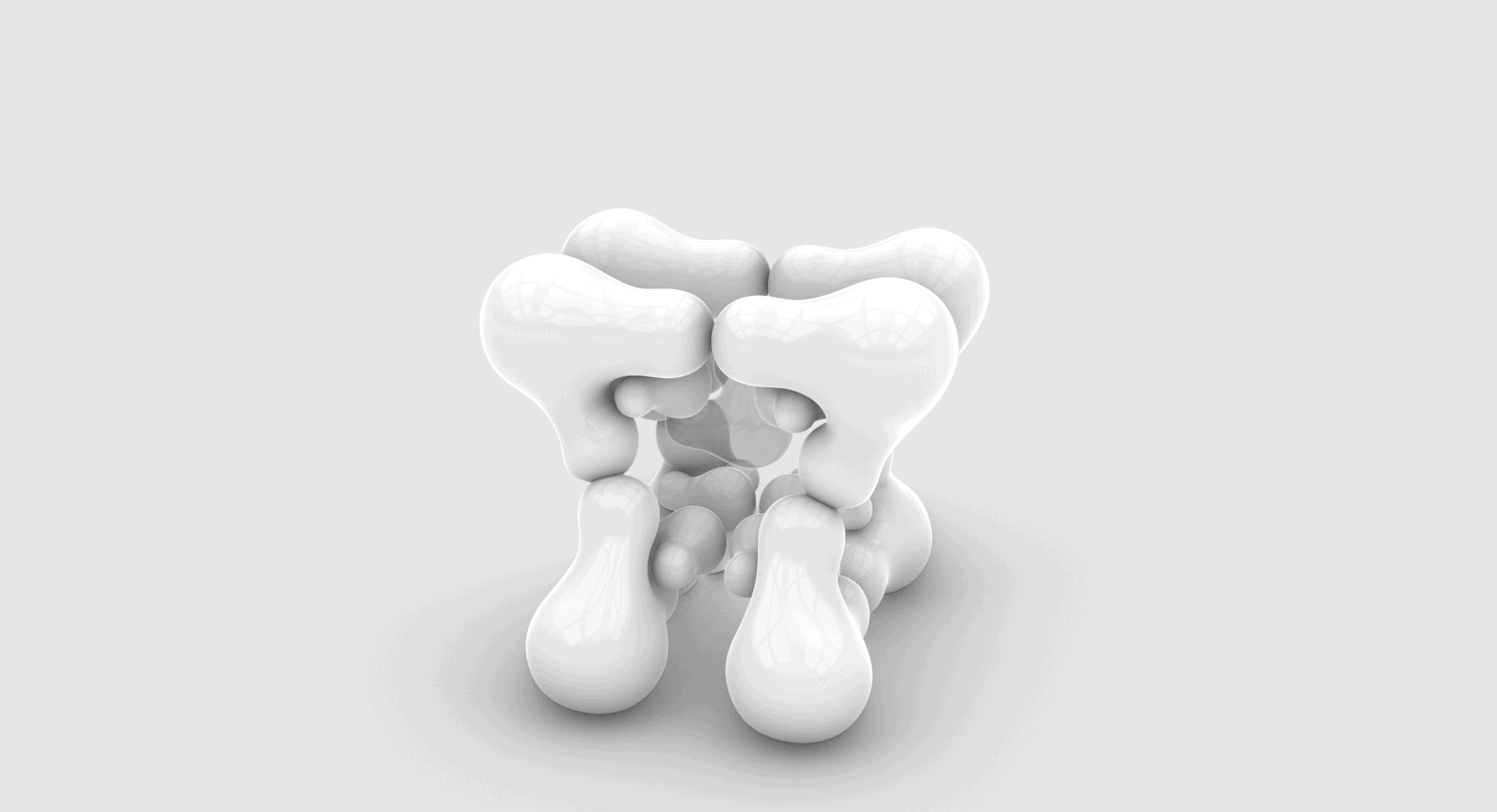
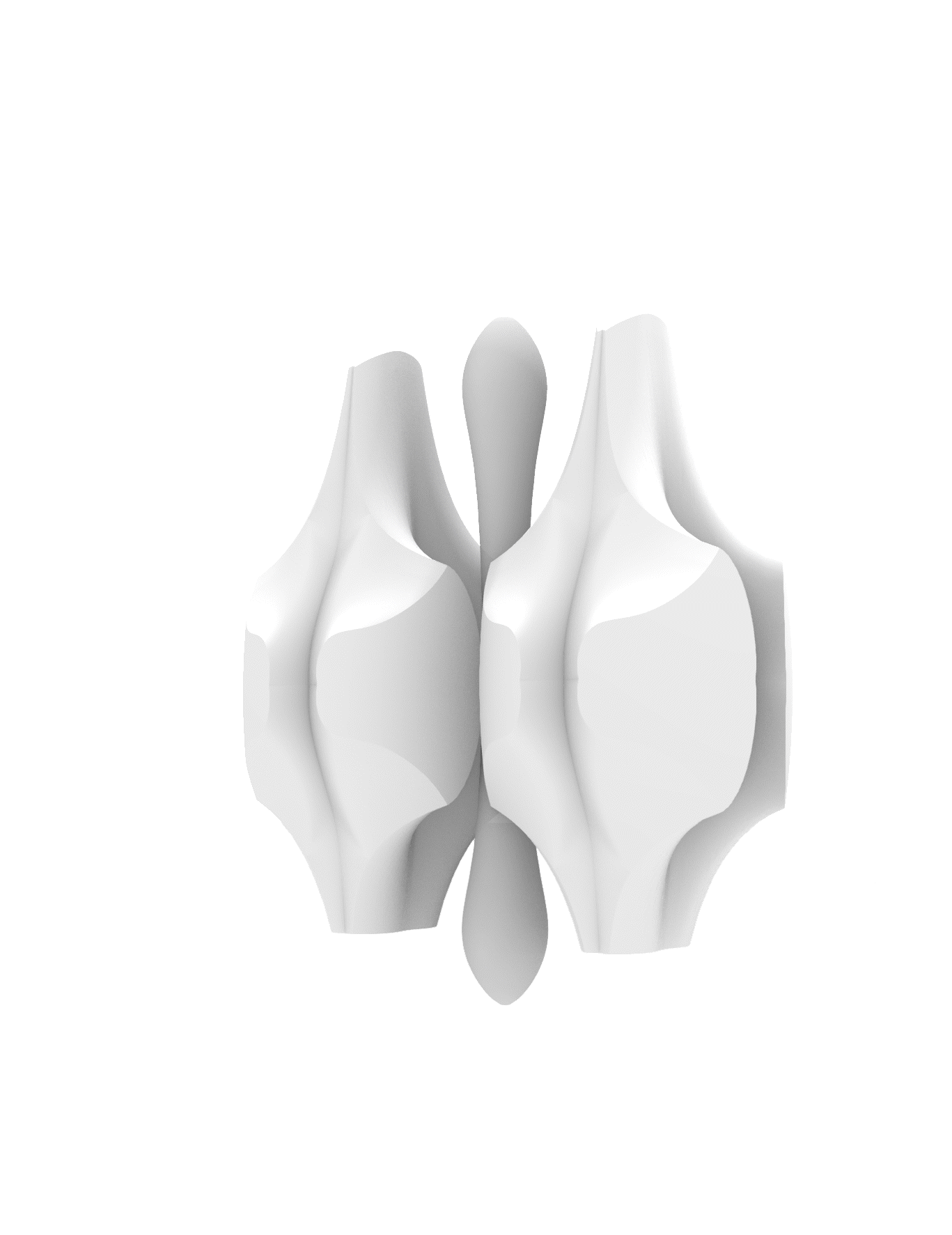
Master of Science in Design: Robotics and Autonomous Systems (MSD-RAS)
Weiztman School of Design, University of Pennsylvania
ARCH 802 Material Agencies III_ Spring Semester
Instructors: Robert Stuart-Smith & Nathan King & Laia Mogas-Soldevila & Jeffrey Anderson
TAs: Mahsa Masalegoo & Franklin Wu
MSD-RAS Program Director: Robert Stuart-Smith
ARI Robotics Lab Operators: Nicholas Sideropoulos & Shunta Moriuchi
Team Lobster: BurcuGocen, BeikelRivas, QingyangXu, ZitongRen